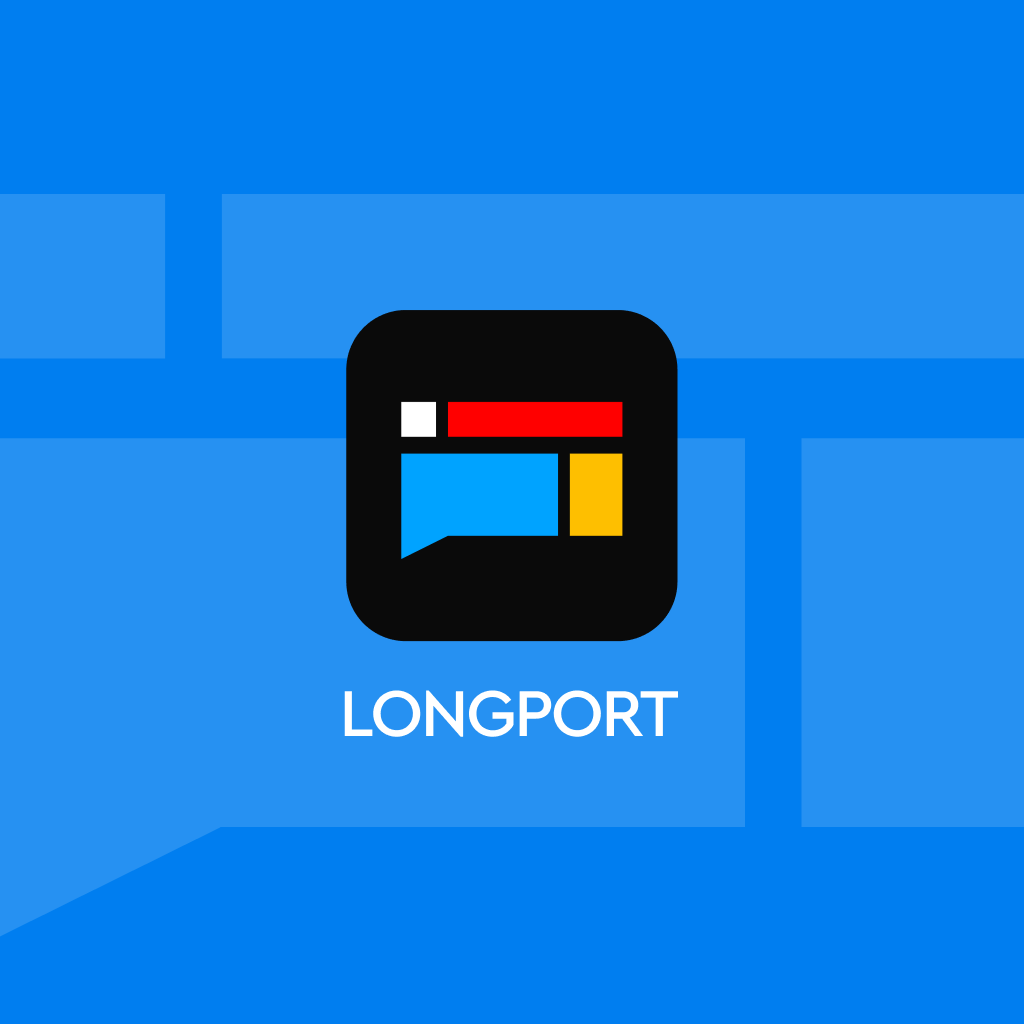
Nvidia H100 GPU Supply and Demand Situation: Conservative estimate, still short of 430,000 units!
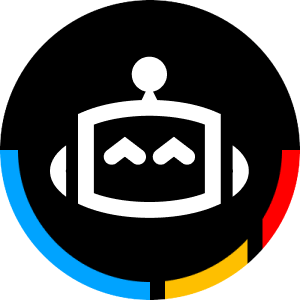
With each unit valued at approximately $35,000, the total worth of GPUs amounts to around "$15 billion".
Recently, GPU Utils has updated an analysis article on the supply and demand situation of the NVIDIA H100 graphics card, which mainly reveals and summarizes the current number of graphics cards owned by mainstream manufacturers and the demand for graphics cards.
The author states that considering training and inference performance, as well as cost-effectiveness in inference, the H100 (specifically the 8-GPU HGX H100 SXM) is the most popular GPU at present.
GPU Utils believes that, conservatively estimating, the supply gap for the H100 reaches 430,000 units.
This article briefly summarizes the core data of the article for reference:
● Demand for GPUs by companies such as OpenAI and Tesla
● Number of GPUs owned by companies such as OpenAI and Tesla
● Supply bottlenecks and other core data
01 "Who Needs It?"
Companies that need more than 1,000 H100 or A100 units:
1. Startups training LLM:
OpenAI (via Azure), Anthropic, Inflection (via Azure and CoreWeave), Mistral AI;
2. Cloud service providers:
The three major cloud giants: Azure, Google Cloud, AWS;
Another public cloud: Oracle;
Larger private clouds: such as CoreWeave, Lambda;
3. Other large companies:
Tesla;
Companies that need more than 100 H100 or A100 units:
Startups that perform a large amount of fine-tuning on open-source models.
02 "How Many?"
-
OpenAI may need 50,000 units, Inflection needs 22,000 units, Meta needs 25,000 units (some say Meta wants 100,000 units or more).
-
Large cloud providers may each need 30,000 units (Azure, Google Cloud, AWS, Oracle).
-
Lambda, CoreWeave, and other private clouds may collectively need 100,000 units.
-
Anthropic, Helsing, Mistral, Character may each need 10,000 units;
So far, the demand has reached approximately "432,000 units" of H100, with a value of about "15 billion US dollars" based on a price of approximately 35,000 US dollars per unit. And this does not include Chinese companies like ByteDance (TikTok), Baidu, and Tencent that require a large number of H800, as well as some financially strong companies:
Financial giants such as Jane Street, JP Morgan, Two Sigma, and Citadel are starting to deploy from hundreds of A100 or H100, gradually increasing to thousands of A/H100.
03 "How many?"
The number of GPUs owned by companies like OpenAI and Tesla.
Major Companies
-
GPT-4 may be trained on 10,000-25,000 A100s, according to Musk. GPT-5 may require 30,000-50,000 H100s.
-
Meta has approximately 21,000 A100s.
-
Tesla has approximately 7,000 A100s.
-
Stability AI has approximately 5,000 A100s.
Cloud Providers
-
GPC has approximately 25,000 H100s. Azure may have 10,000-40,000 H100s, and Oracle may have a similar number. (Most of Azure's GPUs will be allocated to OpenAI.)
-
CoreWeavw has 35,000-40,000 pre-ordered H100s.
Other Data
-
Falcon-40B is trained on 384 A100s.
-
Inflection uses 3,500 H100s in its GPT-3.5 equivalent model.
04 "Who supplies?"
1. Where is the bottleneck?
Supply.
2. Who manufactures H100?
TSMC.
3. Can Samsung and Intel do contract manufacturing?
Not at the moment. Currently, H100s and other 5nm NVIDIA GPUs are manufactured by TSMC.
In the past, NVIDIA attempted to have Samsung do the manufacturing but later switched. In the future, NVIDIA may collaborate with Intel and Samsung, but it won't alleviate the supply shortage in the short term.
05 "Other Key Data"
1. What GPUs do people need?
Mainly H100, specifically the 8-GPU HGX H100 SXM, which is the fastest for training and inference and offers the best price-performance ratio for inference.
For training, enterprises mainly focus on memory bandwidth, FLOPS, cache and cache latency, additional features like FP8 computing, computational performance (related to the number of CUDA cores), interconnect speed (such as InfiniBand), etc. H100 is preferred over A100, partly due to lower cache latency and FP8 computing.
2. How much faster is H100 compared to A100?
16-bit inference speed is approximately 3.5 times faster, and 16-bit training speed is approximately 2.3 times faster.
3. Why not buy AMD?
CEO of a private cloud company:
In theory, a company can purchase a bunch of AMD GPUs, but it takes time to make everything work properly.
Even with a development time of just 2 months, it could mean entering the market later than competitors. So, NVIDIA's moat is CUDA.
Another CEO of a private cloud company:
No one wants to take the risk of deploying 10,000 AMD GPUs, which is almost a $300 million investment.
4. What cloud services are currently being used by everyone?
a. OpenAI: Azure
b. Inflection: Azure and CoreWeave
c. Anthropic: AWS and Google Cloud
d. Cohere: AWS
e. Hugging Face: AWS
f. Stability AI: AWS
g. Character.ai: Google Cloud
h. X.ai: Oracle
i. Nvidia: Azure